Let’s learn about Python Dataframes
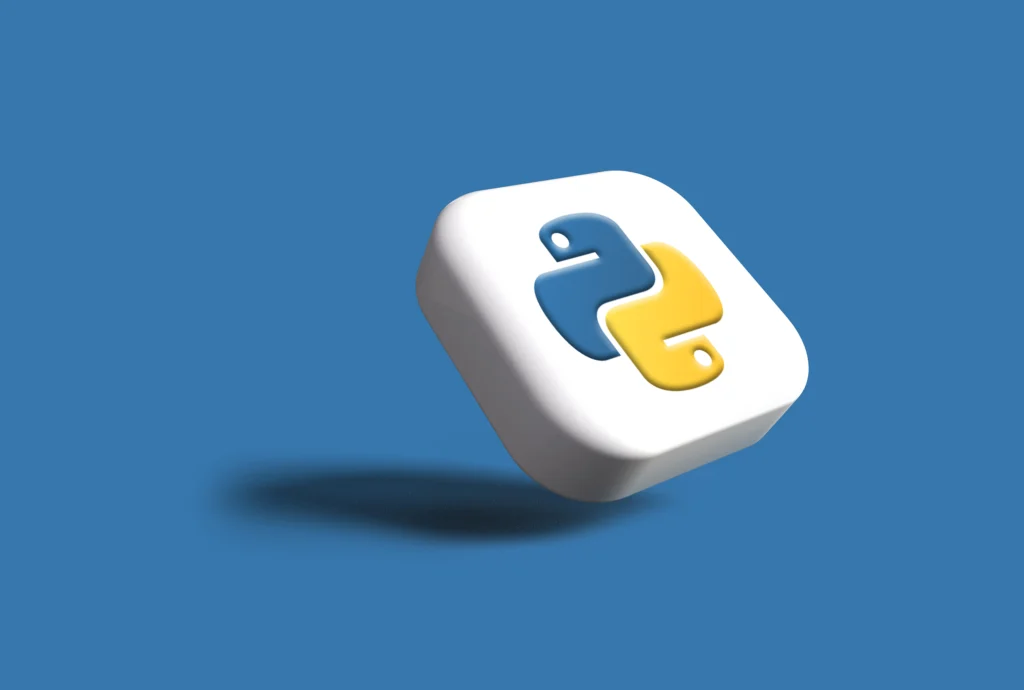
A DataFrame is a 2D, tabular data structure from the pandas library, a cornerstone of data manipulation and analysis in Python. Resembling spreadsheets or SQL tables, DataFrames provide a structured and intuitive approach to organizing and analyzing data. Each column in a Python DataFrame represents a variable, while each row corresponds to a specific observation.
Creating a Python DataFrame
import pandas as pd
# Creating a DataFrame from a dictionary
data = {'Name': ['Alice', 'Bob', 'Charlie'],
'Age': [25, 30, 22],
'City': ['New York', 'San Francisco', 'Los Angeles']}
df = pd.DataFrame(data)
print(df)
Output:
Name Age City
0 Alice 25 New York
1 Bob 30 San Francisco
2 Charlie 22 Los Angeles
Basic Operations with DataFrames:
Manipulating and analyzing data becomes seamless with DataFrames. Fundamental operations include selecting and filtering data, handling missing values, and grouping and aggregating data.
Basic DataFrame Operations
Example 1:
# Selecting a specific column
ages = df['Age']
Output:
0 25
1 30
2 22
Name: Age, dtype: int64
Example 2:
# Filtering data based on a condition
young_people = df[df['Age'] < 30]
Output:
Name Age City
0 Alice 25 New York
2 Charlie 22 Los Angeles
Example 3:
# Handling missing values
df.fillna(0, inplace=True)
Explanation:
This code fills any missing values in the DataFrame with 0. Since the provided DataFrame does not have any missing values, there won’t be a noticeable change in the output. The inplace=True
parameter modifies the original DataFrame.
Related: Python Lists Guide
Example 4:
# Grouping and aggregating data
average_age_by_city = df.groupby('City')['Age'].mean()
Output:
City
Los Angeles 22.0
New York 25.0
San Francisco 30.0
Name: Age, dtype: float64
Indexing and Slicing:
Efficiently extracting subsets of data is crucial. DataFrames support both label-based and position-based indexing and slicing.
# Selecting a row by label
alice_info = df.loc[0]
print("Row by Label - Alice's Information:")
print(alice_info)
print("----------------")
# Slicing rows and columns
subset = df.loc[1:2, ['Name', 'City']]
print("Subset of DataFrame - Rows 1 to 2, Columns 'Name' and 'City':")
print(subset)
Output:
Row by Label - Alice's Information:
Name Alice
Age 25
City New York
Name: 0, dtype: object
----------------
Subset of DataFrame - Rows 1 to 2, Columns 'Name' and 'City':
Name City
1 Bob San Francisco
2 Charlie Los Angeles
Merging and Concatenating DataFrames:
In real-world scenarios, data is often scattered across multiple sources. DataFrames allow seamless merging or concatenation of datasets.
Merging DataFrames
# Creating another DataFrame
data2 = {'Name': ['David', 'Eve'],
'Age': [28, 35],
'City': ['Chicago', 'Seattle']}
df2 = pd.DataFrame(data2)
# Merging DataFrames based on a common column
merged_df = pd.merge(df, df2, on='City')
print("Original DataFrame:")
print(df)
print("----------------")
print("DataFrame to be merged:")
print(df2)
print("----------------")
print("Merged DataFrame:")
print(merged_df)
Output:
Original DataFrame:
Name Age City
0 Alice 25 New York
1 Bob 30 San Francisco
2 Charlie 22 Los Angeles
----------------
DataFrame to be merged:
Name Age City
0 David 28 Chicago
1 Eve 35 Seattle
----------------
Merged DataFrame:
Name_x Age_x City Name_y Age_y
0 Alice 25 New York NaN NaN
1 Bob 30 San Francisco NaN NaN
2 Charlie 22 Los Angeles NaN NaN
3 NaN NaN Chicago David 28.0
4 NaN NaN Seattle Eve 35.0
Advanced Topics:
Delving into advanced topics, we explore reshaping and pivoting data, handling time series data, and using custom functions with DataFrames.
Reshaping Data
# Reshaping data using the melt function
melted_df = pd.melt(df, id_vars=['Name'], value_vars=['Age', 'City'])
print("Original DataFrame:")
print(df)
print("----------------")
print("Melted DataFrame:")
print(melted_df)
Output:
Original DataFrame:
Name Age City
0 Alice 25 New York
1 Bob 30 San Francisco
2 Charlie 22 Los Angeles
----------------
Melted DataFrame:
Name variable value
0 Alice Age 25
1 Bob Age 30
2 Charlie Age 22
3 Alice City New York
4 Bob City San Francisco
5 Charlie City Los Angeles
You might Like: For loops in python
Conclusion:
As we conclude our exploration of Python DataFrames, their indispensable role in data manipulation and analysis becomes evident. The flexibility, efficiency, and extensive functionality of DataFrames make them a cornerstone of data workflows. Mastering the art of working with DataFrames unlocks Python’s full potential for deriving insights and making informed decisions in the world of data science.
Talha is a seasoned Software Engineer with a passion for exploring the ever-evolving world of technology. With a strong foundation in Python and expertise in web development, web scraping, and machine learning, he loves to unravel the intricacies of the digital landscape. Talha loves to write content on this platform for sharing insights, tutorials, and updates on coding, development, and the latest tech trends